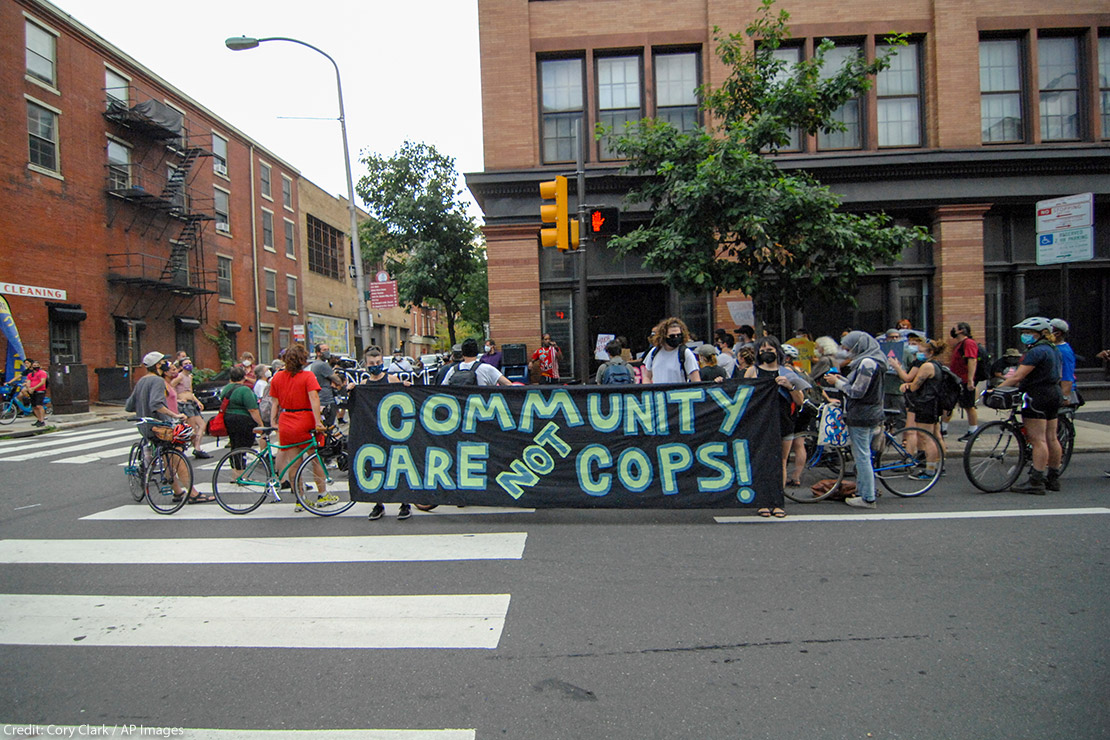
Employers today rely on various kinds of artificial intelligence (AI) or other automated tools in their hiring processes, including to advertise job opportunities, screen applications, assess candidates, and conduct interviews. Many of these tools carry well-documented risks of discrimination that can exacerbate existing inequities in the workplace. Employers should avoid tools altogether that carry a high risk of discrimination based on disabilities, race, sex and other protected characteristics, such as personality assessments and AI-analyzed interviews. But where an employer is considering using or is already using an AI tool, robust auditing for discrimination and other harms is one critical step to address the dangers that these tools pose and ensure that it is not violating civil rights laws.
But as usual, the devil is in the details.
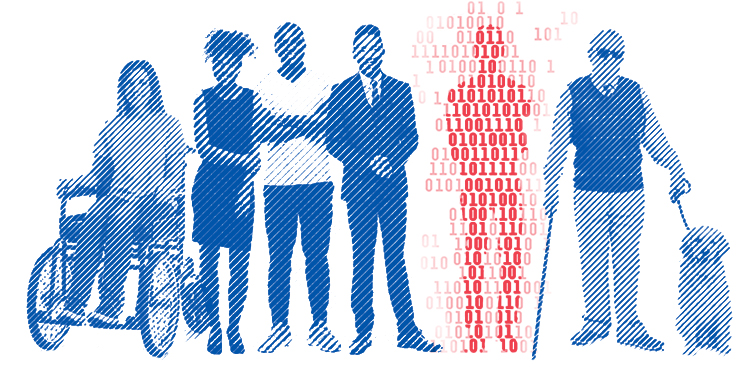
Know Your Rights | Know Your Digital Rights: Digital Discrimination in Hiring
Learn more about how automated tools are used in the hiring process and your digital rights under these laws.
Source: American Civil Liberties Union
A rigorous and holistic discrimination audit of an automated tool — both before and periodically after deployment — can provide employers information to help them determine whether to adopt a tool at all, what mitigation measures may be needed, and whether they need to abandon a tool after adoption. Auditing can also bring much needed transparency when audits are shared with the public, providing critical information for job applicants, researchers, and regulators. On the other hand, algorithm audits that are not carefully crafted can be gamed to present a misleading picture of the system in question or can serve as a cursory box-checking exercise, potentially legitimizing systems that may be discriminatory.
As regulators and legislators are increasingly focused on addressing the impacts of automated systems in critical areas like hiring and employment, including creating requirements for auditing, these efforts must be carefully crafted to ensure that the audits increase accountability in practice. While there is no one-size-fits-all approach to algorithm auditing, audits for bias and discrimination should:
- Evaluate the system’s performance using carefully selected metrics — metrics that consider both when the system works and when it fails.
- Break down performance for people in different groups, including but not limited to race, sex, age, and disability status, and the intersections of those groups.
- Use data that faithfully represents how the system is used in practice.
- Be conducted by auditors who are independent from the entity that built or deployed the algorithm.
In many cases, audits can and should be conducted by interdisciplinary teams of subject matter experts, including social scientists, lawyers and policy researchers, that consult with people who will be impacted by these tools and the users of the system itself. Researchers and practitioners have created many different resources describing how these kinds of audits can be operationalized.
Why the details of algorithm audits are so critical
Examining emerging “bias audits” produced in connection with a recently enacted law in New York City (Local Law 144) helps demonstrate why these details are so critical. Because of this law, employers using some of these kinds of technologies are required to publish “bias audits” with statistics about how often job applicants advance in the hiring process when an automated tool is used, broken down for people of different races and sexes.
Some news coverage has described this law as requiring employers to “prove their AI hiring software isn’t sexist or racist.” But a closer look at these “bias audits” indicates that they are incomplete evaluations of bias and discrimination. First, the auditing requirement only applies to a limited set of the types of automated tools used in hiring processes today. So far, we’ve only been able to locate around a dozen bias audits — even though 99 percent of Fortune 500 companies reportedly use some type of automated system in their hiring processes. The law also doesn’t require the audits to assess possible biases related to many characteristics where discrimination in hiring and employment has long been a concern, including disability, age, and pregnancy.
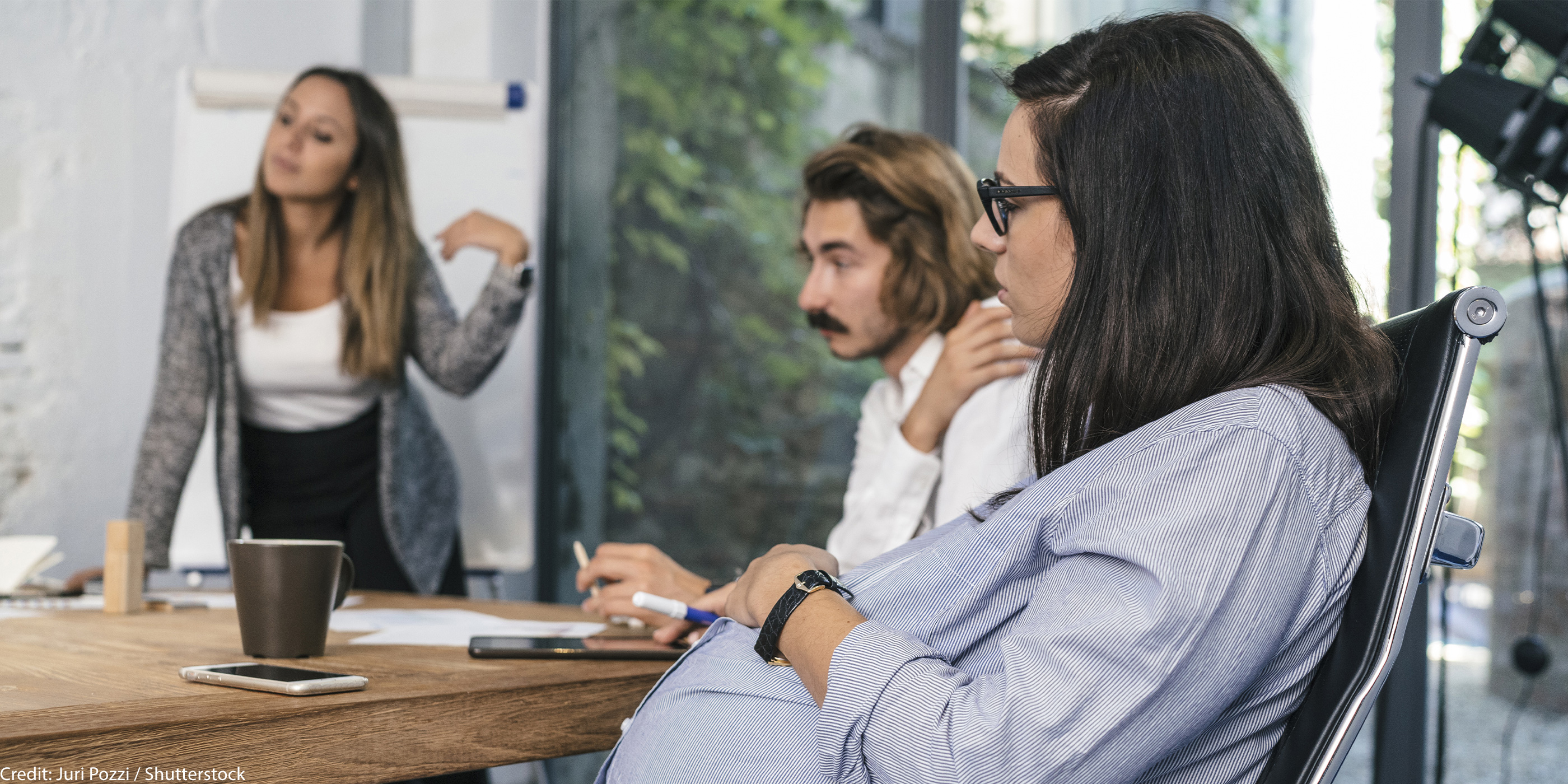
The Historic New Law Protecting Fairness for Pregnant Workers
After a decade of advocacy, the Pregnant Workers Fairness Act is finally going into effect.
Source: American Civil Liberties Union
When it comes to what’s in the audits, the statistics required to be calculated and reported can provide some basic information about which automated tools employers are using in their hiring processes and the number of job applications being evaluated by these tools. But these audits fall short of meaningful transparency in several ways. For one example, some of the audits we’ve seen so far don’t even provide the name or vendor of the tool being audited. The audits also don’t examine whether the tools work as advertised or whether they accurately assess the relevant skills or capabilities needed for a job. In addition, these bias audits may not fully portray the experiences of candidates or practices of employers for multiple reasons. Several of the audits, including this one of an AI-driven candidate screening tool and this one of an AI-driven applicant scoring tool are missing a lot of data on candidates who were evaluated by the automated tool in question.
The published audits also frequently rely on data that is pooled together from multiple employers that use the same tool, even though they may be using the tool in very different ways. Companies characterize these audits as designed to “ensure non-discrimination against protected groups,” when in fact this data pooling may mask stark disparities or discriminatory practices by employers.
More generally, algorithm audits should be publicly available and easy to access as a matter of transparency. Even though employers are required to publish the audits on their websites, so far, we’ve found it quite difficult to locate these bias audits. That’s why we worked with the New York Civil Liberties Union to create a public tracker of all the ones we’ve seen so far (if you know of Local Law 144 bias audits that employers have posted that we missed, let us know by emailing analytics_inquiry@aclu.org).
As automated systems become more entrenched in every part of our lives, audits of these systems can be crucial to identifying and preventing their harms. But for that to be the case, algorithm audits must be holistic, ongoing, and reflective of the ways automated systems are used in practice. Technologists, civil rights advocates, policymakers, and interdisciplinary researchers should work together to ensure that algorithm audits live up to their potential.
Comments
Post a Comment